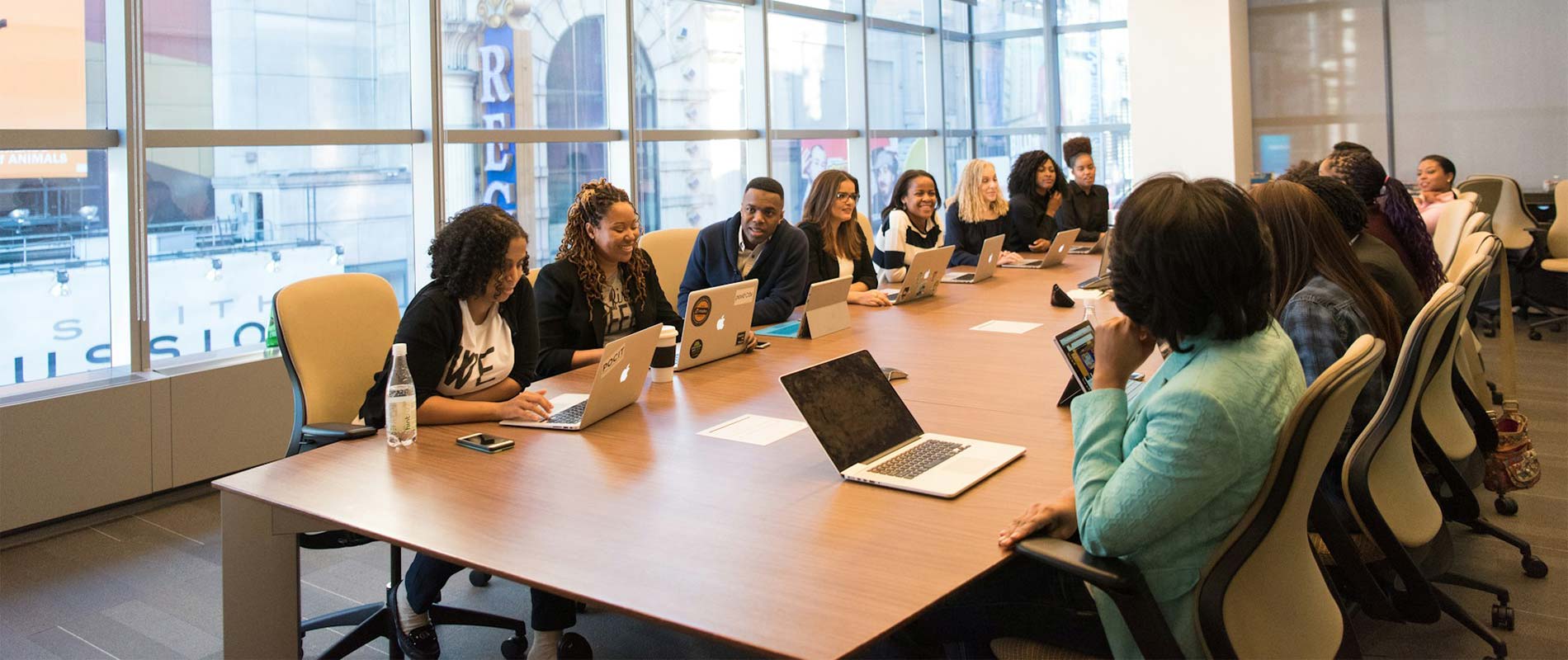
Unifying Your Data Estate for Analytics
Creating powerful insights from a single source of truth.
Organizations today are increasingly recognizing the necessity of investing in advanced digital transformation to leverage the power of data and analytics. However, this journey is riddled with challenges. A common hurdle is the existence of disparate and siloed data systems that suffer from a lack of interoperability. This situation is compounded by the technical nature of platforms, which necessitate advanced analytics expertise that is not always readily available within the organization. As a result, gaining widespread Business Intelligence (BI) adoption becomes a formidable task, which in turn hampers the seamless sharing of data across various teams and lines of business (LOBs). Additionally, the integration of these systems can incur significant costs and require extensive ongoing maintenance, making the process cumbersome for many. With the increase in data volume comes an escalation in security risks that must be carefully managed. Lastly, while there is a pressing need to fulfill the promise of analytics, organizations often find their resources stretched thin, struggling to meet these complex demands.
Today’s Data Value Creation Challenges:
Delivering on the promise of analytics with limited resources
Technical platforms requiring advanced skills
Costly integration and ongoing maintenance
Siloed systems and
data
Gaining BI adoption to streamline data sharing
Data and security
risks
The starting point for many organizations is what’s known as an organically evolved data estate. While the idea of an organically evolved data estate might appear advantageous at first, it often leads to numerous inefficiencies and increased risks of data exposure. These issues can disrupt the connectedness within the organization’s data systems and significantly diminish the chances of achieving successful outcomes.
When an organization grapples with multiple copies of the same data and infrastructural inefficiencies, the implications for its teams are significant. Initially, there are increased costs associated with maintaining and managing these redundant data copies. Secondly, the presence of inaccurate data can lead to challenges in decision-making, as the reliability of the information comes into question. Lastly, the complexity of systems that are overly technical can make them difficult for team members to utilize effectively, further compounding the challenges faced by the organization.
Limited interoperability within an organization can have a detrimental effect on its financial health. It often results in slower and less efficient business operations, as staff must rely on manual data transfers and inefficient workarounds to accomplish tasks that could otherwise be automated. Furthermore, organizations are compelled to invest in custom integrations or middleware solutions to facilitate system connections. These solutions can be not only expensive to develop but also to maintain over time. Additionally, the lack of interoperability can cause organizations to miss out on valuable opportunities to embrace new technologies and services, which could otherwise drive innovation and growth.
In addition to the detrimental impact that limited interoperability can have on a business’s bottom line, the risks associated with data exposure can have serious repercussions for any organization. Data exposure risks include the potential theft or compromise of sensitive or confidential information, which can lead to significant security breaches. Such breaches can result in non-compliance with various regulations, incurring legal consequences and hefty fines. Perhaps most damaging of all is the erosion of trust that can occur among customers, partners, and stakeholders, which can take considerable time and resources to rebuild. This decrease in trust can have long-lasting effects on the reputation and credibility of a business.
Evolving Your Data Estate
The ultimate objective of integrating your hybrid and multi-cloud data estate is to enable every member of your organization to utilize accurate, certified, real-time data to generate more impactful insights. This is achieved by merging data from various disparate sources into a consolidated single source of truth. By doing so, your team can maximize the value of your data investments through an analytics platform capable of connecting to and analyzing data from on-premises, cloud-based, and third-party sources. Adopting a lake-first approach simplifies the storage of vast quantities of diverse data types while concurrently reducing operational costs. To ensure uniform data access, the data is virtualized into an open Lakehouse foundation, which provides a common dataset for everyone. Additionally, this unified system allows for the rapid development of analytics solutions with minimal setup and deployment time, further enhancing the organization’s efficiency and agility.
The Finish Line
From current state to future state. Unifying your hybrid and multi-cloud environments is critical for resilient business transformation and for optimizing the business value that data & analytics can provide. What does this look like?
From: | To: |
Fragmented, compartmentalized, and siloed cloud environments | An analytics platform that connects to & can analyze all your on-prem, cloud-based, and third-party data sources |
High operational costs due to high data storage processing | Lakehouse approach that makes it easier and more efficient to store data |
Siloed data access issues | Data virtualizing in an open Lakehouse to ensure everyone has access to the same data sets |
Complex, slow-to-ramp, and lagging analytics solutions | Spin up analytics solutions quickly with minimal set-up, deployment, and latency |
How To Approach Unifying Your Data Estate
To combat the challenges we previously discussed, there are three data estate architectures and concepts that organizations are applying to the modernization of their data estate. The data mesh, data fabric, and data hub.
Most popular among these frameworks is data mesh, which focuses on building domains that allow LOBs to access the data they need and operate autonomously to build their own data products. While data mesh meets the requirements for LOB users, it can create extra work for the teams responsible for getting data to different domains. By applying key principles of the second framework, data fabric, organizations can implement different data services and add automation to ingest, standardize, curate, and integrate data. This helps deliver data to the domains and accelerate data value creation. The third framework, data hub, provides the foundation to store and secure data, while allowing different tenants to be implemented and aligned to each data domain. Organizations use a data hub to consolidate data sources and apply uniform governance to store all types of data at vary.
By implementing a standardized approach to managing data, organizations can ensure that their data is consistent, accurate, and reliable, making it easier to analyze and derive insights from it.
Data Mesh
A series of domains assigned to individual LOBs that enables access to the data they need with maximum autonomy by upholding the four principles of a data mesh.
Data Fabric
A system for automating data management tasks, such as unifying and cleaning disparate sources as well as authorizing data access, that helps a business make the most of its existing data sources without needing to migrate them.
Data Hub
An open and governed lakehouse platform that provides storage foundation for serving data to multiple domains efficiently, so domain users can easily consume it.
These modern data architectures are not mutually exclusive. But rather are collectively transformative . While there is copious academic debate surrounding these modern architectures, the reality is the combination of them will drive the best solution.
This approach is based on best practices, refined by Microsoft, over more than two decades of experience in building global-scale products and services. It starts with a holistic view of the organization, factoring in the people, processes, culture, and technology. Then, by applying data governance, security, and compliance across every layer of the stack, it ensures organizations get a truly innovative environment that empowers everyone to do their best work.
By 2025, 95% of decisions that currently use data will be at least partially automated.
Source: Gartner, “Striving to Become a Data-Driven Organization? Start with 5 Key D&A Initiatives.”
The Solution: Establishing a Storage Foundation
At a high level, an opened and governed storage foundation allows you to unify your data estate by integrating data from different data sources including on-premises and multi-cloud locations. And the keywords here are open and governed because everything in the data lakehouse analytics foundation is based on open data formats and open data standards. This enables solution durability that’s not possible with other closed-standards or closed-format-based analytics foundations.
Many organizations today use data that resides in a proprietary storage format for their analytics use cases which causes data silos and inefficiencies to form. For example, proprietary data warehouse platforms only serve data for descriptive analytics. That means other analytics practices, such as machine learning, can’t use that data without first extracting it to a data lake which is both time consuming and costly. A lakehouse solution solves this problem by storing data once and serving it to all types of analytics including but not limited to business intelligence, machine learning, streaming analytics, and data exchange. This approach, provides the most cost-effective storage and enables domain teams to easily share data.
This overall approach is based on an open and governed data lakehouse foundation for analytics. The open and governed data lakehouse foundation is a cost-effective and performance-optimized fabric for business intelligence, machine learning, and AI workloads at any scale. It is the foundation for migrating and modernizing existing analytics solutions, whether this be data appliances or traditional data warehouses. Finally, the data lakehouse is foundational for integrating data across the broad spectrum of emerging operational databases and systems including modern analytics applications.
This approach helps organizations both modernize and migrate their existing analytics estate. Doing so achieves maximum cost and performance efficiencies while implementing an open and governed data lakehouse foundation. Implementing the “lake first” pattern is the initial step towards this goal. In the “lake first” pattern, data from all data sources, whether legacy fabrics being migrated or operational systems in production, is first ingested to a data lake on Azure Data Lake Storage Gen 2 (ADLS Gen 2). ADLS Gen 2 is a cost- and performance-optimized data lake storage service for the most demanding Business Intelligence (BI), Machine Learning (ML), and Artificial Intelligence (AI) workloads.
Microsoft even offers comprehensive data migration and integration solutions to enable the lake first pattern. Their solutions include first party services as well as fully integrated third-party services to migrate and integrate data from across a broad spectrum of legacy analytics systems and production systems. Azure Data Factory connectors (now also available in Synapse as pipelines) enable integrating data from a broad spectrum of Microsoft and third-party data fabrics. Azure Synapse Link connectors enable “no code” and “always synchronized” data integration for operational databases in Azure Cosmos DB, Microsoft Dataverse, and SQL—both on-premises with SQL Server 2022 and in the cloud with Azure SQL Database.
By unifying your hybrid multi-cloud enterprise analytics, you’re enabling your lines of business by providing self-serve analytics that empower LOBs to implement their own analytics projects while also democratizing data and analytics. You’ll also be able to re-use data products across domains, reducing data engineering and improving data agility. In doing so, you’ll accelerate cross-business unit collaboration.
With the launch of Microsoft Fabric, Microsoft has embraced an open and governed lakehouse as the underlying SaaS storage, standardizing on Delta Parquet format—the same format Azure Databricks customers are using today.
This means Azure Databricks customers can seamlessly integrate with Microsoft Fabric and augment their analytics systems with Generative AI – on top of the same open and governed lakehouse.
And as a first-party offering, Azure Databricks customers will be able to take advantage of a variety of native Azure capabilities and augment their analytics systems with Generative AI – on top of the same open & governed lakehouse – reducing data estate fragmentation.
In Conclusion
As a Microsoft partner, Oakwood’s Data Team understands the intricacies of the Azure ecosystem and has expertise managing complex analytics projects and integration. We can help you harness the value of your data to gain valuable insights.
Let us help you leverage best-of-breed services for capabilities like Data Engineering, Data Warehousing, Real-time Analytics, Business Intelligence, and more—all on an open and governed Data Lakehouse and a common model for data security, governance, and compliance.