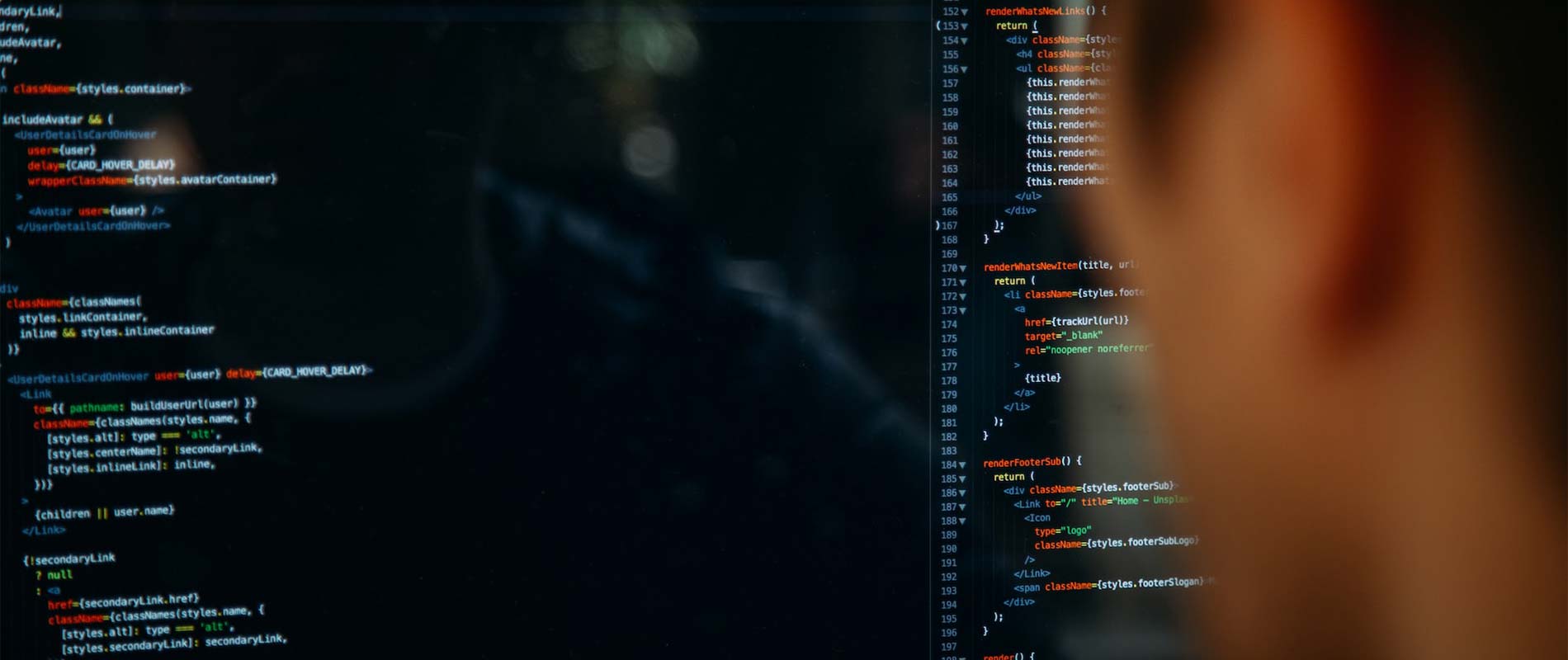
Data Analytics & Machine Learning (ML)
Let our Team of Data Science Consultants help empower your organization in implementing sophisticated Machine Learning models along with a unique AI strategy that fits your long-term business data initiatives.
How Machine Learning Can Impact Your Business
The list of benefits is long. Organizations can optimize operations, shed manual processes, and move faster. In fact, Forbes Magazine stated that 76% of enterprises prioritize AI and machine learning over their other IT initiatives.
We ARE Data Science Experts
We help our clients develop a data-driven culture with insights from their boardroom to the shop floor that delivers faster and easier access to their data and builds intelligence into every process.
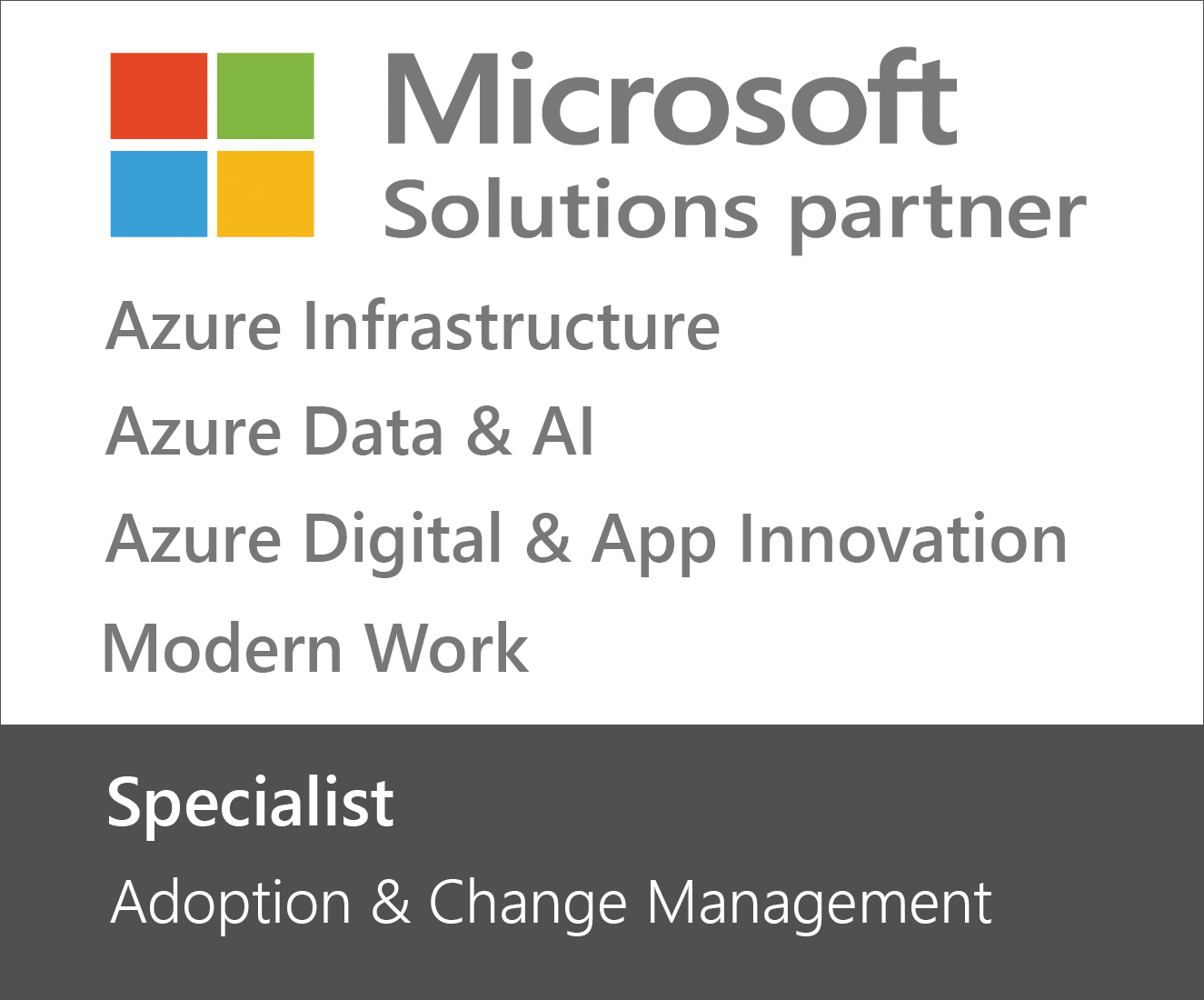
Many organizations travel the data and analytics path to create the technology capabilities, applications, and processes used to collect, store, and analyze data to support business decision making. These organizations are aware of the sheer lift that is involved with setting up the infrastructure, acquiring and retaining personnel, and developing a culture that not only creates data and analytics, but also sustains and develops it.
Most professionals in data and analytics do not view themselves as capable in the domain of Machine Learning. There is a stark line, real or imagined, between Business Intelligence (BI) and Machine Learning (ML). However, advances in machine learning have led to the blurring of this false distinction between BI and ML.
Understanding an organization’s data and analytics capabilities requires an evaluation of where the organization falls on the continuum of a data and analytics evolutionary model (below).
Data & Machine Learning Maturity Model
click below to learn more about your own Data & ML maturity level.
If you’d like to learn more about our capabilities or explore how we can assist in your next Data or Machine Learning initiatives – please leave our Team a note below.