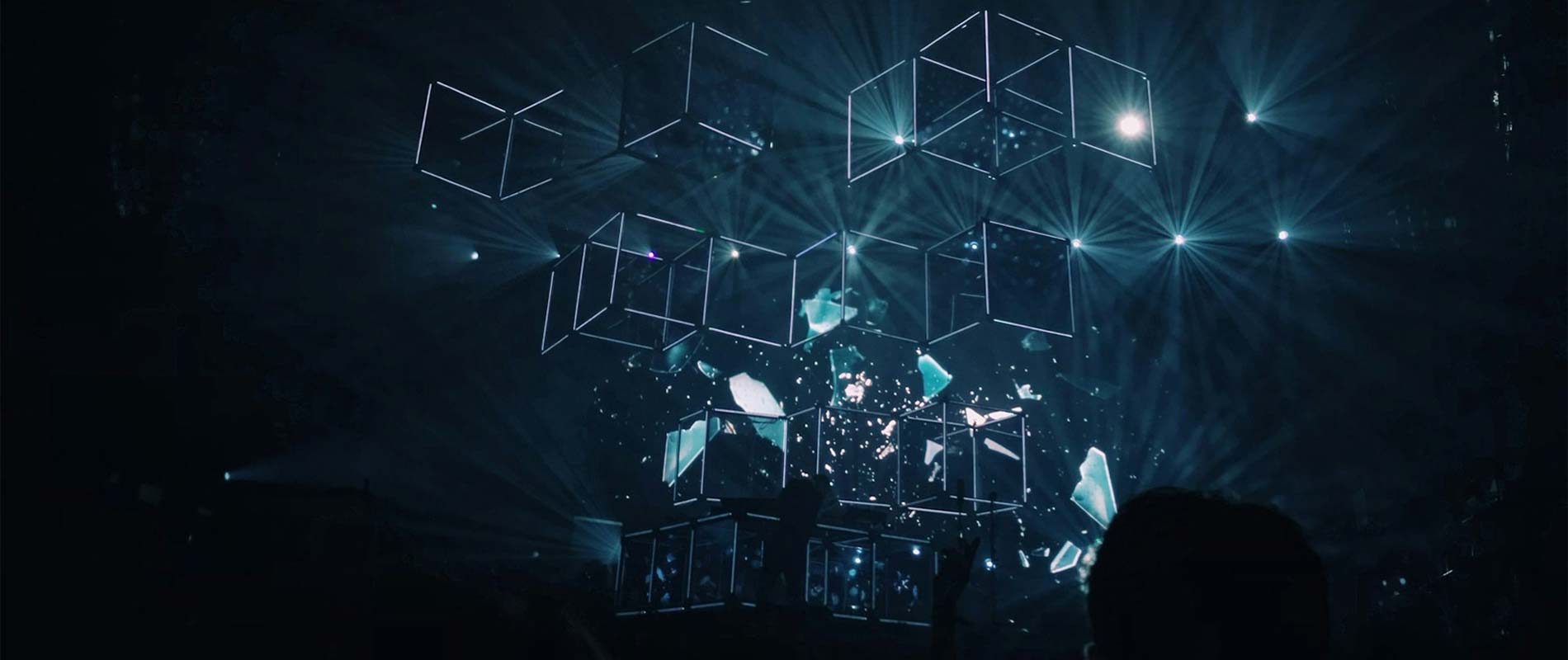
The Crucial Role of Data for AI Readiness
A strong data foundation is pivotal for the success of AI initiatives.
In the ever-evolving landscape of artificial intelligence (AI), organizations, like yours, are racing to harness its transformative potential. However, before leaping into AI implementation, it’s essential to address a fundamental aspect: data readiness. A strong data foundation is pivotal for the success of AI initiatives. Last week, we wrote about the importance of moving infrastructure to Azure as a foundation. Today, we dive deeper to explore the critical components of data readiness, including cloud-based data for OpenAI and Copilot services, data security and governance, and the importance of a modern data estate.
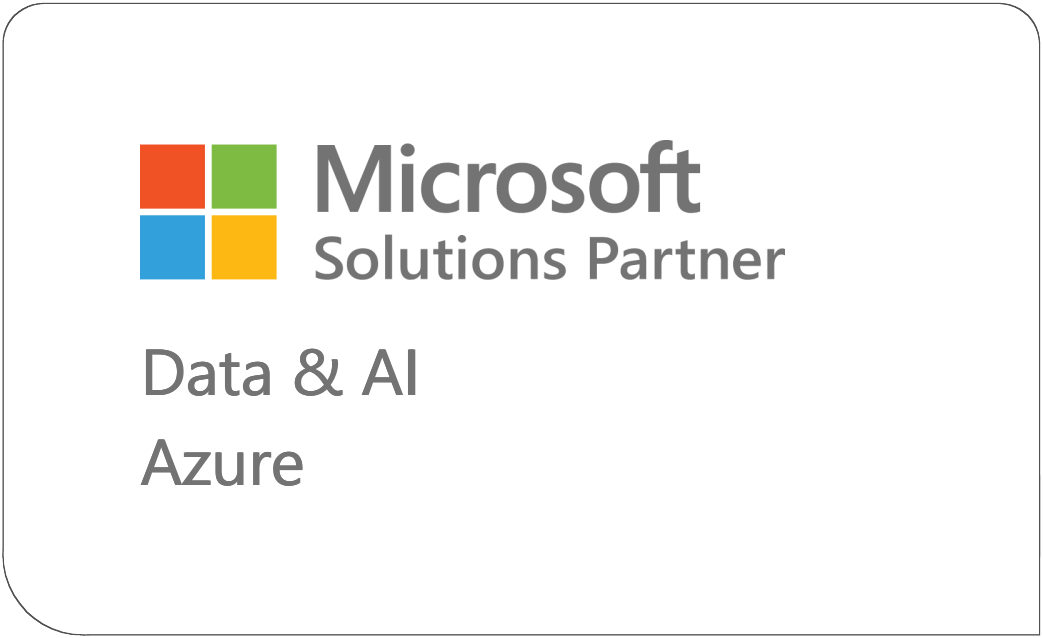
We ARE Data & AI Experts
Our Team has deep expertise in Microsoft technologies and a proven track record in Data & AI solutions, ensuring projects are executed with precision. This certification signifies high competency in data management, AI model development, and integration with Azure’s AI tools, ensuring secure, scalable, and compliant AI implementations that maximize ROI and drive transformative business outcomes.
The Importance of Data Readiness
AI systems are only as good as the data they learn from. The quality, organization, and reliability of your data can make or break your AI projects. Poor data quality leads to inaccurate predictions and flawed insights, undermining the very goals AI is meant to achieve. Conversely, high-quality, well-organized data enhances the performance of AI models, enabling them to deliver valuable and actionable insights. Efficiently organized data not only accelerates the training and deployment of AI models but also reduces time-to-market, giving organizations a competitive edge. Furthermore, robust data governance ensures compliance with regulatory standards, safeguarding organizations from legal risks and maintaining stakeholder trust.
Cloud-Based Data for OpenAI and Copilot Services
Migrating data to the cloud is a significant step toward data readiness, especially for leveraging advanced AI services like OpenAI and Microsoft Copilot. Cloud platforms offer numerous benefits for AI initiatives. Scalability is a primary advantage, allowing organizations to store vast amounts of data necessary for training large AI models. Cloud storage ensures data is easily accessible from anywhere, facilitating seamless collaboration and integration with AI services. Additionally, cloud platforms like Microsoft Azure for AI provides built-in tools and services that streamline the process of deploying AI models, enhancing efficiency and reducing complexity.
Data Security and Governance
With great data comes great responsibility. Ensuring data security and governance is paramount when preparing for AI implementation. Protecting sensitive information from unauthorized access is critical, and this requires implementing encryption, access controls, and anonymization techniques. Data privacy measures not only protect the integrity of the data but also build trust with customers and stakeholders. Compliance with industry regulations and standards, such as GDPR, HIPAA, and CCPA, is essential to avoid legal penalties and maintain customer trust. Implementing robust data governance policies ensures that data is managed consistently and transparently, supporting both operational needs and compliance requirements. Data lineage, which tracks the origin and movement of data within your organization, helps maintain data integrity and ensures adherence to governance policies.
Building a Modern Data Estate
A modern data estate or data warehouse is the backbone of data readiness. It enables efficient data storage, management, and analysis, providing a solid foundation for AI initiatives. A unified data platform consolidates data from disparate sources into a single, consistent repository, ensuring data consistency and accessibility. Data lakes, which store unstructured and semi-structured data, complement traditional data warehouses, enabling comprehensive analysis and AI model training. Advanced analytics and business intelligence tools allow organizations to extract actionable insights from their data, driving informed decision-making and strategic planning. Real-time data processing capabilities provide up-to-date information for AI models and decision-making processes, enhancing responsiveness and agility.
A modern data estate should be designed to support the specific needs of AI and advanced analytics. This includes implementing robust data integration and ETL (Extract, Transform, Load) processes to ensure data from various sources is accurately and consistently ingested into the data estate. Additionally, a modern data estate should support diverse data types and formats, enabling the organization to leverage all available data for AI initiatives. High-performance storage and computing infrastructure are also critical, providing the necessary resources to handle large-scale data processing and analysis tasks.
How To Achieve Data Readiness?
Achieving data readiness involves several strategic steps. The journey begins with a thorough data assessment, evaluating the current state of your data and identifying gaps in quality, organization, and security. This assessment provides a clear understanding of the challenges and opportunities, guiding the subsequent steps in the data readiness process. Data cleansing is a critical step, involving the identification and correction of inaccuracies and redundancies in the data. This process ensures that the data used for AI training and analysis is accurate and reliable.
Migrating data to a cloud platform is another key step in achieving data readiness. Cloud migration not only provides scalability and accessibility benefits but also enables organizations to leverage advanced AI tools and services available on cloud platforms like Microsoft Azure. During the migration process, it is essential to implement robust data security measures, ensuring that sensitive information is protected from unauthorized access. Establishing stringent data governance policies is also crucial, providing a framework for managing and protecting data throughout its lifecycle.
Modernizing your data estate is the final step in achieving data readiness. This involves building or upgrading your data warehouse to support advanced analytics and real-time processing. A modern data estate should provide the necessary infrastructure and tools to store, manage, and analyze large volumes of data, enabling organizations to unlock the full potential of AI.
Technical Considerations for Data Readiness
When preparing for AI implementation, several technical considerations must be addressed to ensure data readiness. One critical aspect is data integration, which involves consolidating data from various sources into a unified platform. This requires implementing robust ETL processes to extract, transform, and load data into the data estate. Ensuring data quality throughout the integration process is essential, as poor-quality data can significantly impact the performance of AI models.
Another important consideration is data storage. A modern data estate should provide scalable and high-performance storage solutions, capable of handling large volumes of structured and unstructured data. Cloud storage solutions, such as those offered by Microsoft Azure, provide the necessary scalability and performance, enabling organizations to efficiently store and manage their data. Additionally, data storage solutions should support diverse data types and formats, enabling organizations to leverage all available data for AI initiatives.
Data security and governance are also critical technical considerations. Implementing robust security measures, such as encryption, access controls, and data masking, ensures that sensitive information is protected from unauthorized access. Establishing comprehensive data governance policies provides a framework for managing data throughout its lifecycle, ensuring compliance with regulatory standards and maintaining data integrity. Additionally, implementing data lineage capabilities allows organizations to track the origin and movement of data, supporting both operational and compliance needs.
Finally, building a modern data estate requires high-performance computing infrastructure. AI and advanced analytics tasks often involve processing large volumes of data, requiring significant computational resources. Cloud platforms like Microsoft Azure provide scalable and high-performance computing infrastructure, enabling organizations to efficiently handle data processing and analysis tasks. Additionally, leveraging advanced analytics and business intelligence tools allows organizations to extract actionable insights from their data, driving informed decision-making and strategic planning.
Conclusion
As organizations prepare for AI adoption, data readiness emerges as a critical factor for success. Ensuring your data is clean, well-organized, and reliable lays a solid foundation for AI initiatives. Leveraging cloud-based data storage, implementing stringent data security and governance measures, and building a modern data estate are essential steps toward achieving data readiness. At Oakwood, our Data & AI Team is here to guide you through this transformative journey, ensuring your data is ready to unlock the full potential of AI. By addressing the technical and strategic aspects of data readiness, organizations can confidently embark on their own AI journey, driving innovation and achieving a sustainable competitive advantage.